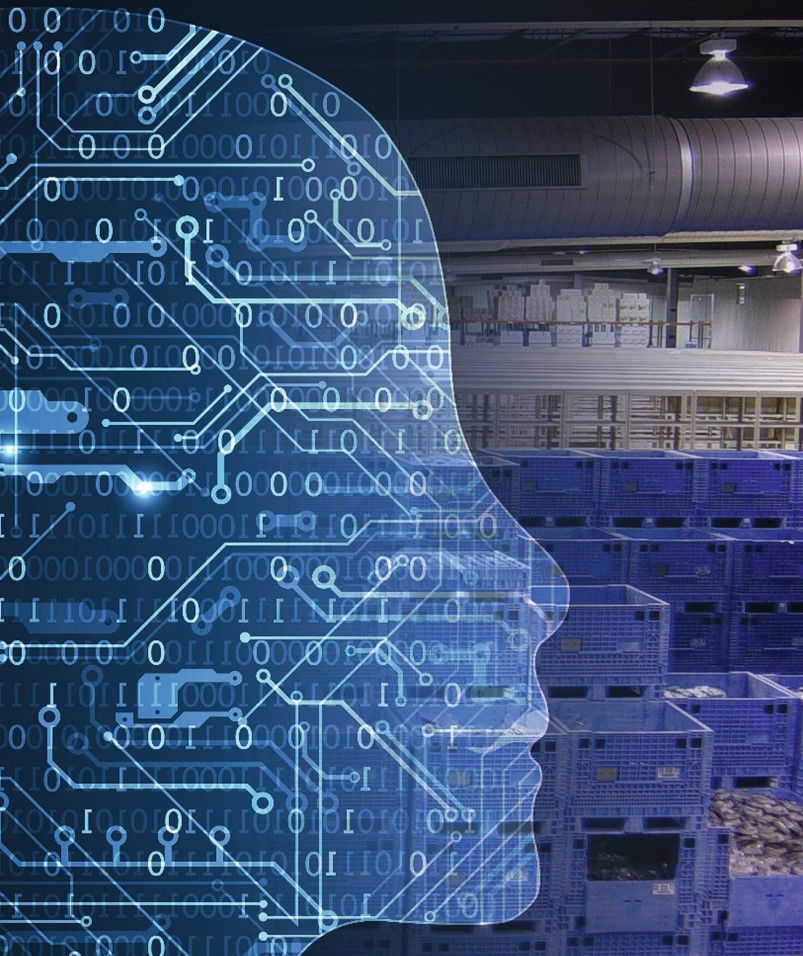
From hype to practice: Reacting was yesterday – machine learning in container management
Containers play an important role as a link between production steps. Due to ongoing automation and clearly defined packaging regulations, the use of reusable load carriers is essential. The establishment of the lean philosophy reinforces this fact by avoiding repackaging processes, among other things. As a result, reusable containers as the smallest logistical unit have become an indispensable part of the production logistics process. Their availability is therefore an elementary factor for the company’s success. Due to the ongoing globalization and the associated multitude of exchange relationships, this is not an easy task.
There are many container management systems (BMS) that are based on the use of analytical models and can offer the container manager a new basis and support for decision-making through the targeted and controlled extraction of information and knowledge from existing data. The existing data is used both in the BMS to control the container cycles (inventory management, push/pull supply of SC participants) and for long-term planning and loop optimization (shifting the order source, dimensioning the container cycle).
But are these measures sufficient for the requirements of tomorrow?
In the everyday life of the container manager, it is increasingly the case that long-term planning meets short-term reality. Planning is only relevant as long as the framework conditions remain constant. This is less and less the case in tomorrow’s – and even today’s – world. Instead, the conditions are unstable and therefore unpredictable. The static framework for previous low-risk planning is becoming increasingly blurred.
Machine learning concepts can be seen as an important tool to supplement analytical models for data analysis. Artificial intelligence can help to turn previous “bold” planning and perhaps rather rough assumptions into valid container management in the short term. This also makes it possible to react quickly to changes, as these can be detected at an early stage, for example through anomaly detection. These technologies are forward-looking and are both a driver and a goal within the current paradigm shift in logistics towards the data-driven business models of tomorrow – and they are urgently needed.
More than just a brief acquaintance: LOGSOL and AI get to know each other better
LOGSOL has joined forces with the Technical University of Dresden in a joint project to develop methods for applying machine learning algorithms using analytical and simulative models for container management. The aim of the joint efforts is to use the possibilities of digitalization to master and profitably exploit the associated challenges with regard to the extensive data basis. Ultimately, a container management system can be offered that provides users with the best possible support and enables them to make the right decisions.
Of particular importance here is the area of predictive analytics, which refers to the generation of reliable forecasts from current and historical data. In container management, the forecasting of container bookings creates a basis on which a variety of assistance functions can be built for container owners. Using this knowledge, they are able to plan their stocks optimally even in the event of dynamic changes to the general conditions and thus, for example, strategically delegate orders when bottlenecks occur. Without such assistance and decision-making support, the decisions made by dispatchers are and remain subject to a high degree of uncertainty and therefore an increased risk.
In container management, the challenge of using forecasting models such as neural networks, support vector machines or decision trees lies in the dependency of the container flow on the underlying production process. It requires an in-depth analysis of the data basis in order to be able to identify data with actual “explanatory power”, which is able to forecast, for example, the expected outgoing bookings for the coming week with sufficient accuracy.
The use cases of machine learning in container management successfully tested in the project range from
- the forecasting of bookings and stock
- the use of classification, clustering and forecasting models to evaluate account partners and suppliers
- the use of sentiment analysis and text evaluation tools to improve the response to system users’ concerns.
For this purpose, a suitable model is selected from a set of training data and configured on the basis of parameter variations. A KPI trend is then predicted for validation by including multivariate influencing variables using the ML-based forecast and compared with the actual reference data. This data can then be displayed in a dashboard for decision support.
Data is silver, information is gold
Continuous testing of artificial intelligence technologies is very important for the logistics industry. However, it also presents companies with many challenges, as it is an interface topic. In addition to domain-specific application and expert knowledge, successful implementation also requires extensive knowledge in the field of data science. While applications using analytical models have already arrived in today’s world, these are no longer sufficient for the applications of tomorrow. In order to be adequately prepared for the future requirements of a “VUCA* world”, decision-intelligent algorithms with machine learning processes are a necessary prerequisite. This means that forecasting container bookings using neural networks is less of an optional extra and is increasingly becoming a must.
*VUCA “Volatility” (“Volatilität”), “Uncertainty” (“Unsicherheit”), “Complexity” (“Komplexität”), “Ambiguity” (“Mehrdeutigkeit”)